Understanding Machine Learning: A Comprehensive Overview
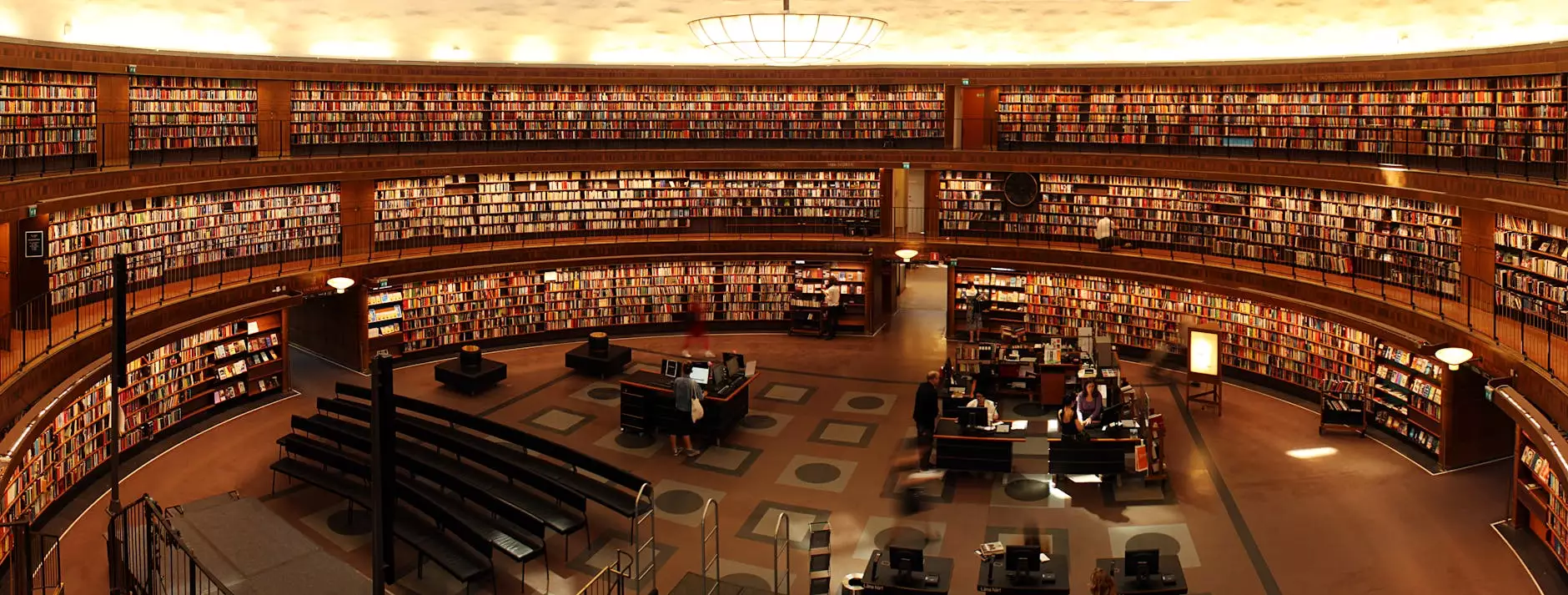
Machine Learning has emerged as one of the most transformative technologies of our time. It is a subfield of artificial intelligence (AI) that focuses on enabling computers to learn from data and improve their performance over time without being explicitly programmed. This article serves as a brief about machine learning and delves into its fundamentals, applications, and implications for businesses across various sectors.
What is Machine Learning?
At its core, machine learning is about creating algorithms that allow computers to identify patterns in large datasets. It equips systems to make predictions or decisions based on those patterns, reducing human intervention. The rapid growth of data generation in recent years has fueled the development of machine learning, providing essential capabilities across numerous applications.
The Evolution of Machine Learning
The roots of machine learning can be traced back to the mid-20th century. Here’s a brief timeline of its evolution:
- 1950s: The concept of machines that can learn from data began to take shape.
- 1980s: With advances in computing power and the introduction of algorithms like backpropagation, neural networks found their way back into research.
- 1990s: The term "machine learning" rose to prominence, with algorithms becoming more sophisticated.
- 2000s: The explosion of the internet and big data catalyzed the widespread use of machine learning in various industries.
Key Concepts in Machine Learning
To fully grasp machine learning, it is essential to understand a few key concepts:
1. Data
Data is the foundation of machine learning. The algorithms learn from *training data*, which consists of examples that help the model understand input-output relationships.
2. Algorithms
Algorithms are sets of rules or instructions that guide the learning process. Common machine learning algorithms include:
- Supervised Learning: This approach involves training a model on labeled data, meaning the outcomes are known.
- Unsupervised Learning: In this method, the model learns from unlabeled data, identifying patterns and groupings within.
- Reinforcement Learning: This technique focuses on training algorithms through trial and error, receiving rewards for correct actions.
3. Features and Labels
Features are individual measurable properties used as input for models, while labels are the output variables the model predicts. For instance...
"In a housing price prediction model, features could include square footage and the number of bedrooms, while the label is the price."
Applications of Machine Learning
Machine learning is being utilized across a myriad of sectors, driving significant advancements and efficiencies. Some key applications include:
1. Healthcare
In the healthcare sector, machine learning algorithms analyze patient data to assist in diagnoses, predict disease outbreaks, and personalize treatment plans. For instance, algorithms can help radiologists identify tumors in medical images with greater accuracy.
2. Finance
In finance, machine learning aids in fraud detection, risk assessment, and algorithmic trading. By analyzing transaction patterns, these systems can flag suspicious activities, ensuring safer financial transactions.
3. Marketing
Businesses leverage machine learning to enhance customer experiences. Targeted advertising, recommended products, and customer segmentation are just some areas benefiting from data-driven insights, allowing for highly personalized marketing strategies.
4. Transportation
Machine learning is integral to the development of autonomous vehicles. It allows vehicles to learn from their surroundings, improving safety and navigation through continuous data assimilation.
Benefits of Machine Learning for Businesses
The integration of machine learning into business operations presents numerous advantages, including:
- Increased Efficiency: Automating processes reduces time spent on mundane tasks, freeing up resources for more critical activities.
- Enhanced Decision-Making: Data-driven insights provide a clearer view of market trends, consumer behavior, and business performance.
- Cost Reduction: Machine learning can lower operational costs through process optimization and predictive maintenance.
- Competitive Advantage: Companies using machine learning can respond to market changes swiftly, positioning themselves ahead of competitors.
Challenges of Machine Learning
Despite its numerous advantages, implementing machine learning comes with challenges. Some common hurdles include:
1. Data Quality
The accuracy of machine learning models depends heavily on data quality. Inaccurate, incomplete, or biased data can lead to ineffective or unfair algorithms. Ensuring high-quality data is paramount.
2. Interpretation of Results
Machine learning models can often appear as "black boxes," making it difficult to interpret how conclusions are reached. This opacity can be problematic, particularly in sectors like healthcare and finance, where understanding decision factors is crucial.
3. Integration with Existing Systems
Incorporating machine learning solutions into existing business processes requires a strategic approach. Companies must be prepared for potential disruptions and invest in training personnel to adapt to new technologies.
The Future of Machine Learning
Looking forward, machine learning is set to revolutionize even more industries. Key trends shaping its future include:
1. Advancements in Deep Learning
Deep learning, a subset of machine learning involving multi-layered neural networks, is becoming increasingly sophisticated. It promises enhanced accuracy in complex tasks such as image and speech recognition.
2. Growth of No-Code and Low-Code Frameworks
As the demand for machine learning solutions grows, so does the development of no-code and low-code platforms, democratizing access to machine learning tools for non-technical users.
3. Ethical AI Development
The conversation around ethical AI is intensifying, focusing on ensuring that machine learning algorithms promote fairness and transparency, minimizing biases and adverse effects on society.
Conclusion
In summary, this article provides a brief about machine learning—its definitions, applications, challenges, and future prospects. As businesses increasingly turn to machine learning to drive growth and innovation, understanding these concepts will be essential for stakeholders in every industry.
Machine learning is not just a technological advancement; it is a fundamental framework changing the way we perceive and interact with data. Its potential is boundless, making it a critical area of focus for businesses looking to thrive in an ever-evolving landscape.