Harnessing Healthcare Datasets for Machine Learning: Unlocking Opportunities in Business Growth
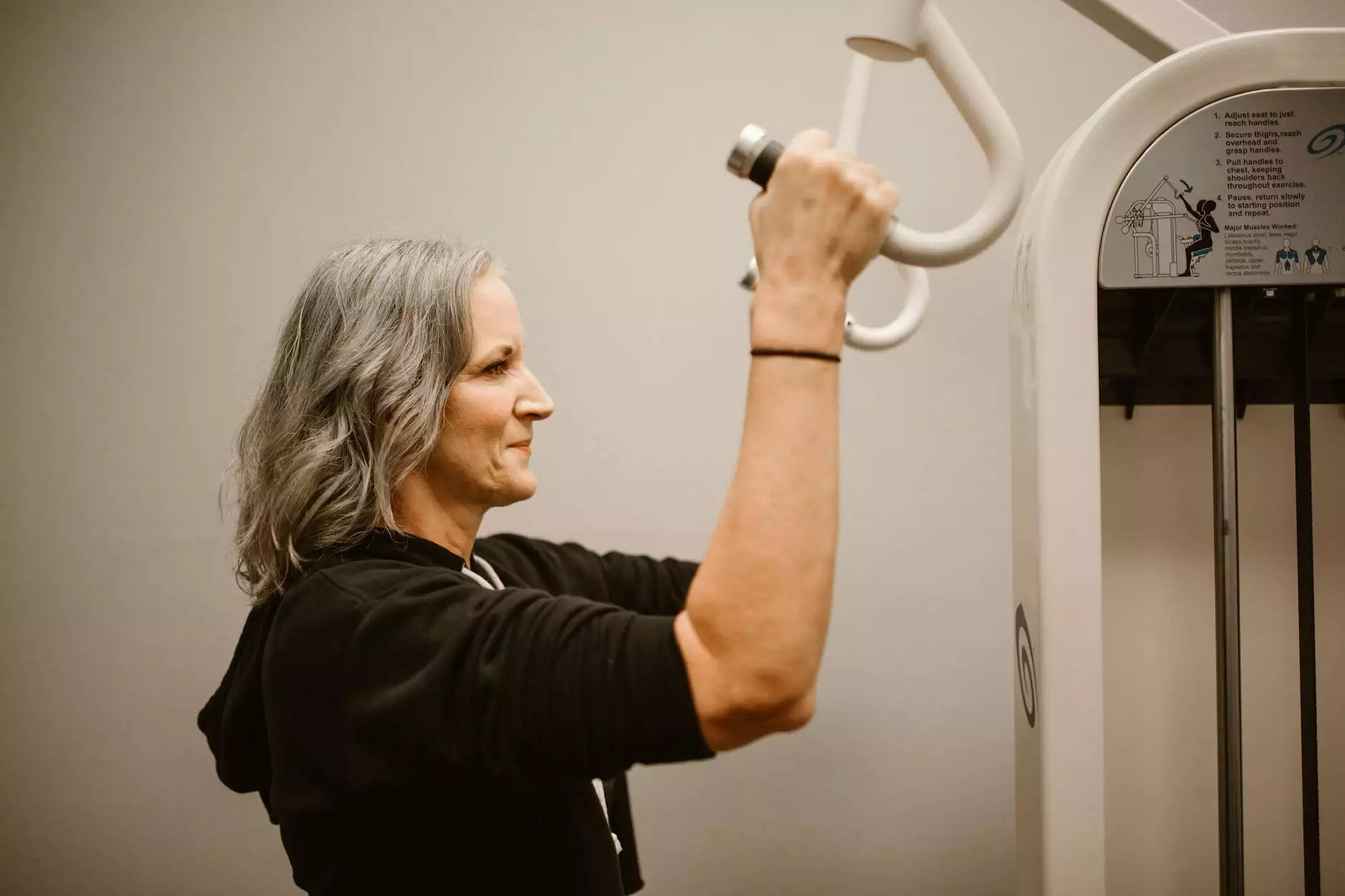
In today's fast-paced digital world, businesses of all shapes and sizes are realizing the enormous potential of healthcare datasets for machine learning. The intersection between healthcare and technology offers immense opportunities, especially for companies seeking to innovate and enhance their service capabilities. By utilizing data-driven methods and advanced analytics, businesses can not only improve performance but also ensure long-term sustainability and growth.
The Impact of Machine Learning on the Healthcare Sector
Machine learning (ML) is revolutionizing the healthcare sector, enabling organizations to draw actionable insights from vast amounts of data. Healthcare datasets for machine learning allow companies to predict trends, enhance patient outcomes, and streamline operational efficiency.
- Improved Patient Care: Machine learning algorithms can analyze patient data to enhance diagnosis accuracy and treatment recommendations.
- Predictive Analytics: By examining historical data, machine learning can identify patterns that help predict future patient conditions, enabling proactive intervention.
- Operational Efficiency: ML can optimize processes like scheduling and resource allocation, ultimately saving time and costs.
Leveraging Healthcare Datasets for Business Growth
Businesses, particularly in the Home Services, Keys & Locksmiths sectors, can leverage healthcare datasets for machine learning to develop intelligent solutions tailored to client needs and operational efficiency.
1. Enhancing Customer Experience
Integrating machine learning into customer service frameworks can significantly enhance customer experience. By analyzing healthcare datasets, locksmiths can identify potential risks and vulnerabilities in homes, allowing them to offer personalized security solutions.
Moreover, understanding customer behavior and preferences through data analysis can help businesses tailor their services. For instance, knowing when customers most frequently require emergency locksmith services can lead to better resource allocation and rapid response times.
2. Predictive Maintenance
ML algorithms can predict when a locking mechanism is likely to fail based on usage patterns and other contributing factors. By utilizing healthcare datasets for machine learning, businesses can extend the lifespan of their products and provide customers with proactive maintenance services.
This predictive maintenance not only reduces repair costs but also increases customer satisfaction as they are less likely to encounter unexpected failures.
3. Data-Driven Decision Making
Incorporating machine learning into decision-making processes enables firms to be more agile and responsive to market changes. By analyzing trends from healthcare datasets, businesses can make informed decisions about resource allocation, service expansion, and consumer engagement strategies.
Challenges in Utilizing Machine Learning and Healthcare Datasets
While the potential for utilizing healthcare datasets for machine learning is significant, businesses face several challenges that must be addressed to fully harness this technology.
- Data Privacy and Security: Handling sensitive healthcare data requires stringent measures to comply with regulations such as HIPAA. Businesses must invest in secure data management systems to protect customer information.
- Data Quality: Ensuring the quality and relevance of healthcare datasets is crucial. Poor-quality data can lead to inaccurate predictions and ineffective solutions.
- Integration with Existing Systems: Incorporating machine learning capabilities into existing business systems can be complex and requires skilled personnel to manage the integration process.
Steps to Implement Machine Learning in Your Business
Implementing machine learning in your business doesn't have to be a Herculean task. By following a structured approach, you can effectively utilize healthcare datasets for machine learning and drive substantial improvements in your services.
1. Define Your Goals
Before diving into machine learning, it’s essential to outline the specific objectives you aim to achieve. These could range from improving customer service to optimizing inventory management.
2. Invest in Data Infrastructure
Your data infrastructure should be robust enough to handle large volumes of healthcare data while ensuring security and compliance. Evaluate existing systems and consider necessary upgrades to facilitate machine learning.
3. Collect and Clean Data
Gather relevant healthcare datasets and ensure they are cleaned and formatted correctly for use. This step is critical as high-quality data leads to better model performance.
4. Choose the Right Algorithms
Select appropriate ML algorithms based on your business objectives. Different algorithms are suited for different types of predictive tasks, so understanding your needs will help narrow down choices.
5. Train and Test Your Model
Once you have selected an algorithm, train it using your datasets. Testing the model's effectiveness with a separate validation dataset will help ensure accuracy before deployment.
6. Implement and Monitor
After successful testing, you can implement the machine learning model into your operational processes. Continue to monitor its performance and make adjustments as needed based on evolving business needs.
Real-World Applications of Machine Learning from Healthcare Datasets
The practical applications of using healthcare datasets for machine learning in business are vast and varied. Here are a few real-world examples:
1. Smart Home Security
Locksmith companies are beginning to use machine learning to enhance smart home security systems. By analyzing behavioral patterns of users, these systems can predict potential security breaches and proactively alert homeowners.
2. Tailored Marketing Strategies
Businesses can analyze healthcare datasets to understand demographic segments better and tailor their marketing strategies accordingly, ensuring they reach the right audience with the right message.
3. Improved Service Delivery
Home service providers can utilize machine learning to streamline their service delivery processes, ensuring timely and efficient responses to customer inquiries and service requests.
Conclusion
The integration of healthcare datasets for machine learning into business operations—especially in sectors like Home Services, Keys, and Locksmiths—is not just a trend; it’s a necessity for companies aiming to survive and thrive in today’s competitive landscape. By harnessing the power of data, businesses can enhance customer experiences, drive operational efficiencies, and position themselves as leaders in their respective markets.
As challenges in data management and algorithm deployment remain, the potential benefits far outweigh the hurdles. Embracing machine learning is a step toward future-proofing your business while delivering unparalleled value to your customers.